Documents
Presentation Slides
Methodical Design and Trimming of Deep Learning Networks: Enhancing External BP learning with Internal Omnipresent-Supervision Training Paradigm
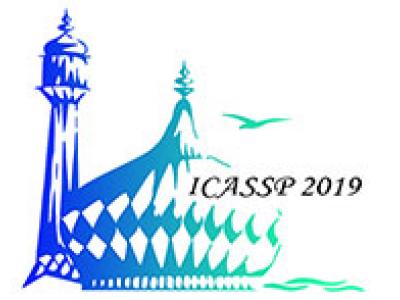
- Citation Author(s):
- Submitted by:
- Zejiang Hou
- Last updated:
- 10 May 2019 - 2:03pm
- Document Type:
- Presentation Slides
- Document Year:
- 2019
- Event:
- Presenters:
- S.Y.Kung
- Paper Code:
- 1897
- Categories:
- Log in to post comments
Back-propagation (BP) is now a classic learning paradigm
whose source of supervision is exclusively from the external
(input/output) nodes. Consequently, BP is easily vulnerable
to curse-of-depth in (very) Deep Learning Networks
(DLNs). This prompts us to advocate Internal Neuron’s
Learnablility (INL) with (1)internal teacher labels (ITL); and
(2)internal optimization metrics (IOM) for evaluating hidden
layers/nodes. Conceptually, INL is a step beyond the notion
of Internal Neuron’s Explainablility (INE), championed by
DARPA’s XAI (or AI3.0). Practically, INL facilitates a structure/
parameter NP-iterative learning for (supervised) deep
compression/quantization: simultaneously trimming hidden
nodes and raising accuracy. Pursuant to our simulations, the
NP-iteration appears to outperform several prominent pruning
methods in the literature.
ICASSP2019.pdf
