Documents
Poster
Poster
NMF-based Comprehensive Latent Factor Learning with Multiview Data
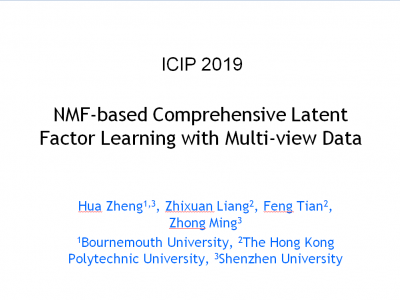
- Citation Author(s):
- Submitted by:
- Hua Zheng
- Last updated:
- 11 September 2019 - 2:26am
- Document Type:
- Poster
- Document Year:
- 2019
- Event:
- Presenters:
- Zhixuan Liang
- Paper Code:
- 3099
- Categories:
- Log in to post comments
Multiview representations reveal the latent information of the data from different perspectives, consistency, and complementarity. Unlike most multiview learning approaches, which focus only one perspective, in this paper, we propose a novel unsupervised multiview learning algorithm, called comprehensive latent factor learning (CLFL), which jointly exploits both consistent and complementary information among multiple views. CLFL adopts a non-negative matrix factorization based formulation to learn the latent factors. It learns the weights of different views automatically which makes the representation more accurate. Experiment results on a synthetic and several real datasets demonstrate the effectiveness of our approach.