Documents
Poster
VISUAL TRACKING VIA SIAMESE NETWORK WITH GLOBAL SIMILARITY
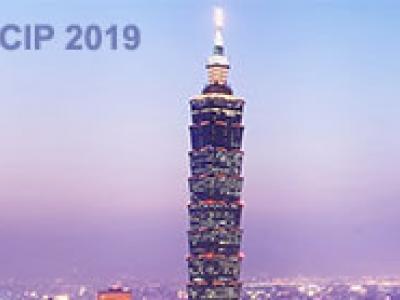
- Citation Author(s):
- Submitted by:
- Fan Chao
- Last updated:
- 16 September 2019 - 5:20am
- Document Type:
- Poster
- Document Year:
- 2019
- Event:
- Presenters:
- Chao Fan
- Paper Code:
- 2119
- Categories:
- Keywords:
- Log in to post comments
Visual tracking is a very important and challenging problem in the field of computer vision. In recent years, Siamese networks have been widely used for visual tracking due to their fast tracking speed, but many trackers based on Siamese network train their networks by utilizing either pairwise loss or triplet loss, which easily leads to over-fitting. In addition, it is difficult to distinguish some hard samples in the training samples. In this paper, we propose a novel global similarity loss to train the network. Specifically, we utilize two Gaussian distributions to simulate and optimize the distribution of positive and negative samples in the train set and add constraint on the hard samples. In experiments, without any other modification, we apply the proposed method to the Siamese network. And the results on several popular tracking benchmarks show our method achieves superior tracking performance than the baseline.