Documents
Poster
Efficient Person Re-Identification in Videos Using Sequence Lazy Greedy Determinantal Point Process (SLGDPP)
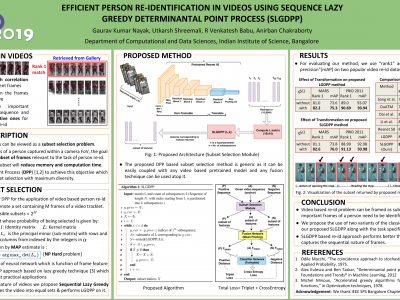
- Citation Author(s):
- Submitted by:
- Gaurav Nayak
- Last updated:
- 19 September 2019 - 6:40am
- Document Type:
- Poster
- Document Year:
- 2019
- Event:
- Presenters:
- GAURAV KUMAR NAYAK
- Paper Code:
- 3410
- Categories:
- Log in to post comments
Given a sequence of observations for each person in each camera, identifying or re-identifying the same person across different cameras is one of the objectives of video surveillance systems. In the case of video based person re-id, the challenge is to handle the high correlation between temporally adjacent frames. The presence of non-informative frames results in high redundancy which needs to be removed for an efficient re-id. We propose a novel method to handle this challenge using Determinantal Point Process (DPP) to select the most diverse and informative subset of frames from a given sequence. Since subset selection problem is NP-Hard, we propose to use an approximate solution called Lazy Greedy DPP (LGDPP) and further extend it to utilize the temporal information of sequences with our proposed Sequential LGDPP (SLGDPP) for video-based person re-id. The major advantages of the proposed DPP variants are their simplicity and plug and play nature, which make it possible to use them atop any pretrained re-id model followed by a feature fusion module. The effectiveness of proposed frameworks is demonstrated on two popular video re-id benchmark datasets through improvements over state-of-the-art methods and naive baseline sampling methods.