Documents
Poster
VAE/WGAN-BASED IMAGE REPRESENTATION LEARNING FOR POSE-PRESERVING SEAMLESS IDENTITY REPLACEMENT IN FACIAL IMAGES
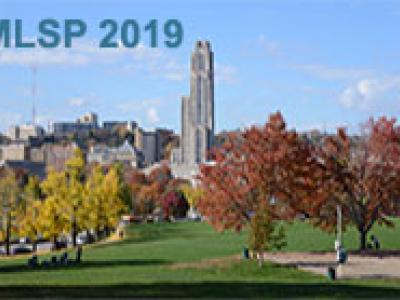
- Citation Author(s):
- Submitted by:
- Hiroki Kawai
- Last updated:
- 11 October 2019 - 4:41pm
- Document Type:
- Poster
- Document Year:
- 2019
- Event:
- Presenters:
- Hiroki Kawai
- Paper Code:
- 56
- Categories:
- Log in to post comments
We present a novel variational generative adversarial network (VGAN) based on Wasserstein loss to learn a latent representation
from a face image that is invariant to identity but preserves head-pose information. This facilitates synthesis of a realistic face
image with the same head pose as a given input image, but with a different identity. One application of this network is in
privacy-sensitive scenarios; after identity replacement in an image, utility, such as head pose, can still
be recovered. Extensive experimental validation on synthetic and real human-face image datasets performed under 3 threat
scenarios confirms the ability of the proposed network to preserve head pose of the input image, mask the input identity,
and synthesize a good-quality realistic face image of a desired identity. We also show that our network can be used to perform
pose-preserving identity morphing and identity-preserving pose morphing. The proposed method improves over a recent state-
of-the-art method in terms of quantitative metrics as well as synthesized image quality.