Documents
Poster
GENERATIVE MODELS FOR LOW-RANK VIDEO REPRESENTATION AND RECONSTRUCTION FROM COMPRESSIVE MEASUREMENTS
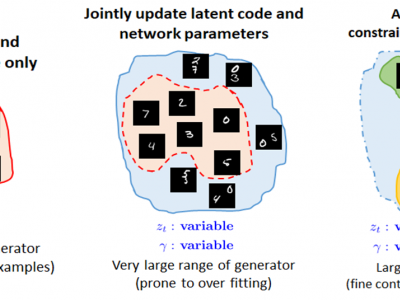
- Citation Author(s):
- Submitted by:
- Rakib Hyder
- Last updated:
- 4 December 2019 - 7:13am
- Document Type:
- Poster
- Document Year:
- 2019
- Event:
- Presenters:
- Rakib Hyder
- Paper Code:
- 214
- Categories:
- Log in to post comments
Generative models have recently received considerable attention in the field of compressive sensing. If an image belongs to the range of a pretrained generative network, we can recover it from its compressive measurements by estimating the underlying compact latent code. In practice, all the pretrained generators have certain range beyond which they fail to generate reliably. Recent researches show that convolutional generative structures are biased to generate natural images. Based on this hypothesis, we propose joint optimization of latent codes and the weights of the generative network in compressive sensing. The main advantage of this method is that we no longer need a pretrained generator as we are optimizing weights of the network. Furthermore, we are getting compact representation of each image from latent code optimization. We empirically demonstrate that our proposed method provides better or comparable accuracy and low complexity compared to the existing methods on different video compressive sensing problems.
For details: https://arxiv.org/pdf/1902.11132.pdf (438)