Documents
Poster
TOWARDS THE DEVELOPMENT OF SUBJECT-INDEPENDENT INVERSE METABOLIC MODELS
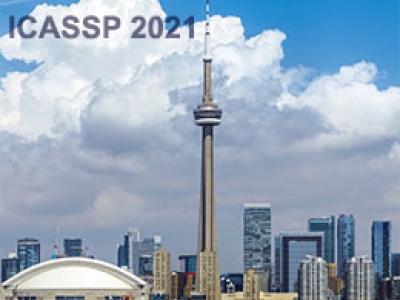
- Citation Author(s):
- Submitted by:
- Seyedhooman Sajjadi
- Last updated:
- 21 June 2021 - 6:15pm
- Document Type:
- Poster
- Document Year:
- 2021
- Event:
- Presenters:
- Seyedhooman Sajjadi
- Paper Code:
- MLSP-43.5
- Categories:
- Log in to post comments
Diet monitoring is an important component of interventions
in type 2 diabetes, but is time-intensive and often inaccurate. To address this issue, we describe an approach to monitor diet automatically, by analyzing fluctuations in glucose
after a meal is consumed. In particular, we evaluate three
standardization techniques (baseline correction, feature normalization, and model personalization) that can be used to
compensate for the large individual differences that exist in
food metabolism. Then, we build machine learning models
to predict the amounts of macronutrients in a meal from the
associated glucose responses. We evaluate the approach on a
dataset containing glucose responses for 15 participants who
consumed 9 meals. Three techniques improve the accuracy
of the models: subtracting the baseline glucose, performing
z-score normalization, and scaling the amount of macronutrients by each individuals’ body mass index