Documents
Presentation Slides
POLA: Online Time Series Prediction by Adaptive Learning Rates
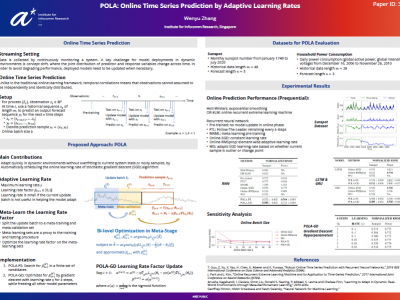
- Citation Author(s):
- Submitted by:
- Wenyu Zhang
- Last updated:
- 22 June 2021 - 1:36am
- Document Type:
- Presentation Slides
- Document Year:
- 2021
- Event:
- Presenters:
- Wenyu Zhang
- Paper Code:
- MLSP-22.6
- Categories:
- Log in to post comments
Online prediction for streaming time series data has practical use for many real-world applications where downstream decisions depend on accurate forecasts for the future. Deployment in dynamic environments requires models to adapt quickly to changing data distributions without overfitting. We propose POLA (Predicting Online by Learning rate Adaptation) to automatically regulate the learning rate of recurrent neural network models to adapt to changing time series patterns across time. POLA meta-learns the learning rate of the stochastic gradient descent (SGD) algorithm by assimilating the prequential or interleaved-test-then-train evaluation scheme for online prediction. We evaluate POLA on two real-world datasets across three commonly-used recurrent neural network models. POLA demonstrates overall comparable or better predictive performance over other online prediction methods.
POLA_slides.pdf

POLA_poster.pdf
