Documents
Poster
End-to-End Audio-Visual Speech Recognition with Conformers
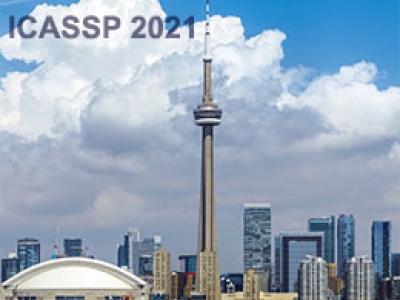
- Citation Author(s):
- Submitted by:
- Pingchuan Ma
- Last updated:
- 22 June 2021 - 4:51am
- Document Type:
- Poster
- Event:
- Presenters:
- Pingchuan Ma
- Paper Code:
- HLT-10.6
- Categories:
- Keywords:
- Log in to post comments
In this work, we present a hybrid CTC/Attention model based on a modified ResNet-18 and Convolution-augmented transformer (Conformer), that can be trained in an end-to-end manner. In particular, the audio and visual encoders learn to extract features directly from raw pixels and audio waveforms, respectively, which are then fed to conformers and then fusion takes place via a Multi-Layer Percep- tron (MLP). The model learns to recognise characters using a com- bination of CTC and an attention mechanism. We show that end-to- end training, instead of using pre-computed visual features which is common in the literature, the use of a conformer, instead of a recur- rent network, and the use of a transformer-based language model, significantly improve the performance of our model. We present results on the largest publicly available datasets for sentence-level speech recognition, Lip Reading Sentences 2 (LRS2) and Lip Read- ing Sentences 3 (LRS3), respectively. The results show that our pro- posed models raise the state-of-the-art performance by a large mar- gin in audio-only, visual-only, and audio-visual experiments.