Documents
Presentation Slides
FULLY-NEURAL APPROACH TO VEHICLE WEIGHING AND STRAIN PREDICTION ON BRIDGES USING WIRELESS ACCELEROMETERS
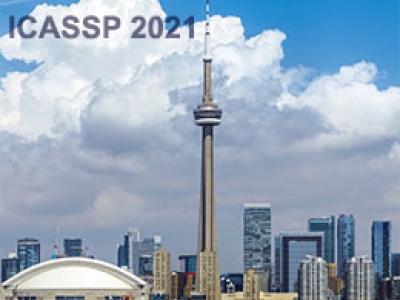
- Citation Author(s):
- Submitted by:
- Takaya Kawakatsu
- Last updated:
- 23 June 2021 - 9:29am
- Document Type:
- Presentation Slides
- Document Year:
- 2021
- Event:
- Presenters:
- Takaya Kawakatsu
- Paper Code:
- ASPS-6.6
- Categories:
- Log in to post comments
Bridge weigh-in-motion (BWIM) is a technique of estimating vehicle loads on bridges and can be used to assess a bridge's structural fatigue and therefore its life.
BWIM can be realized by analyzing the bridge deflection in terms of its response to moving axle loads.
To obtain accurate load estimates, current BWIM systems require strain sensors, whose (re-) installation costs have limited their application.
In this paper, we propose a new BWIM approach based on a deep neural network using accelerometers, which are easier to install than strain sensors, thus helping the advancement of low-cost BWIM systems.
By learning the bridge dynamism, our model estimates axle loads successfully from the noisy acceleration signals sampled on a real bridge in service.
icassp21s.pdf
