Documents
Poster
SINGLE IMAGE SUPER-RESOLUTION VIA GLOBAL-CONTEXT ATTENTION NETWORKS
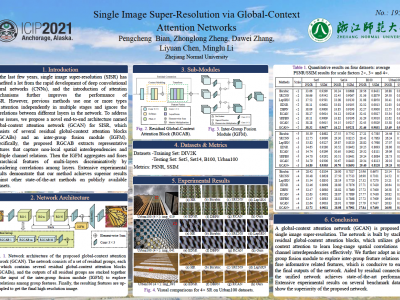
- Citation Author(s):
- Submitted by:
- Pengcheng Bian
- Last updated:
- 25 September 2021 - 2:12am
- Document Type:
- Poster
- Document Year:
- 2021
- Event:
- Presenters:
- Pengcheng Bian
- Paper Code:
- 1950
- Categories:
- Log in to post comments
In the last few years, single image super-resolution (SISR) has benefited a lot from the rapid development of deep convolutional neural networks (CNNs), and the introduction of attention mechanisms further improves the performance of SISR. However, previous methods use one or more types of attention independently in multiple stages and ignore the correlations between different layers in the network. To address these issues, we propose a novel end-to-end architecture named global-context attention network (GCAN) for SISR, which consists of several residual global-context attention blocks (RGCABs) and an inter-group fusion module (IGFM). Specifically, the proposed RGCAB extracts representative features that capture non-local spatial interdependencies and multiple channel relations. Then the IGFM aggregates and fuses hierarchical features of multi-layers discriminatively by considering correlations among layers. Extensive experimental results demonstrate that our method achieves superior results against other state-of-the-art methods on publicly available datasets.
1950_poster.pdf

1950_ICIP21.pdf
