Documents
Poster
DOMAIN-INVARIANT REPRESENTATION LEARNING FROM EEG WITH PRIVATE ENCODERS
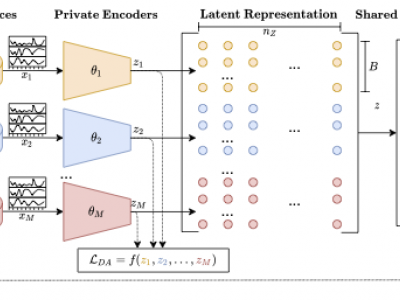
- Citation Author(s):
- Submitted by:
- Philipp Hallgarten
- Last updated:
- 5 May 2022 - 4:03am
- Document Type:
- Poster
- Document Year:
- 2022
- Event:
- Presenters:
- Philipp Hallgarten
- Paper Code:
- 2998
- Categories:
- Log in to post comments
Deep learning based electroencephalography (EEG) signal processing methods are known to suffer from poor test-time generalization due to the changes in data distribution. This becomes a more challenging problem when privacy-preserving representation learning is of interest such as in clinical settings. To that end, we propose a multi-source learning architecture where we extract domain-invariant representations from dataset-specific private encoders. Our model utilizes a maximum-mean-discrepancy (MMD) based domain alignment approach to impose domain-invariance for encoded representations, which outperforms state-of-the-art approaches in EEG-based emotion classification. Furthermore, representations learned in our pipeline preserve domain privacy as dataset-specific private encoding alleviates the need for conventional, centralized EEG-based deep neural network training approaches with shared parameters.