Documents
Presentation Slides
Generalization Ability of MOS Prediction Networks
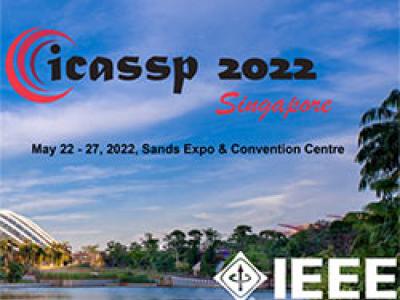
- Citation Author(s):
- Submitted by:
- Erica Cooper
- Last updated:
- 5 May 2022 - 6:00am
- Document Type:
- Presentation Slides
- Document Year:
- 2022
- Event:
- Presenters:
- Erica Cooper
- Paper Code:
- SPE-84.5
- Categories:
- Log in to post comments
Automatic methods to predict listener opinions of synthesized speech remain elusive since listeners, systems being evaluated, characteristics of the speech, and even the instructions given and the rating scale all vary from test to test. While automatic predictors for metrics such as mean opinion score (MOS) can achieve high prediction accuracy on samples from the same test, they typically fail to generalize well to new listening test contexts. In this paper, using a variety of networks for MOS prediction including MOSNet and self-supervised speech models such as wav2vec2, we investigate their performance on data from different listening tests in both zero-shot and fine-tuned settings. We find that wav2vec2 models fine-tuned for MOS prediction have good generalization capability to out-of-domain data even for the most challenging case of utterance-level predictions in the zero-shot setting, and that fine-tuning to in-domain data can improve predictions. We also observe that unseen systems are especially challenging for MOS prediction models.