Documents
Presentation Slides
TRAINING STRATEGIES FOR AUTOMATIC SONG WRITING: A UNIFIED FRAMEWORK PERSPECTIVE
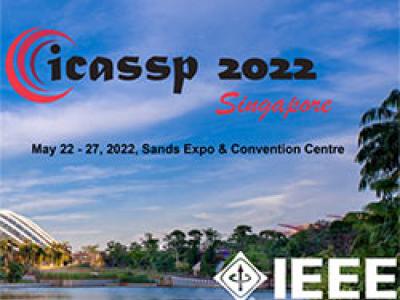
- Citation Author(s):
- Submitted by:
- Tao Qian
- Last updated:
- 5 May 2022 - 7:30am
- Document Type:
- Presentation Slides
- Document Year:
- 2022
- Event:
- Presenters:
- Tao Qian
- Categories:
- Log in to post comments
Automatic song writing (ASW) typically involves four tasks: lyric-to-lyric generation, melody-to-melody generation, lyric-to-melody generation, and melody-to-lyric generation.
Previous works have mainly focused on individual tasks without considering the correlation between them, and thus a unified framework to solve all four tasks has not yet been explored.
In this paper, we propose a unified framework following the pre-training and fine-tuning paradigm to address all four ASW tasks with one model. To alleviate the data scarcity issue of paired lyric-melody data for lyric-to-melody and melody-to-lyric generation, we adopt two pre-training stages with unpaired data. In addition, we introduce a dual transformation loss to fully utilize paired data in the fine-tuning stage to enforce the weak correlation between melody and lyrics.
We also design an objective music generation evaluation metric involving the chromatic rule and a more realistic setting, which removes some strict assumptions adopted in previous works.
To the best of our knowledge, this work is the first to explore ASW for pop songs in Chinese.
Extensive experiments demonstrate the effectiveness of the dual transformation loss and the unified model structure encompassing all four tasks. The experimental results also show that our proposed new evaluation metric aligns better with subjective opinion scores from human listeners.
ICASSP2022.pdf
