Documents
Presentation Slides
Robust Recovery of Jointly-Sparse Signals Using Minimax Concave Loss Function
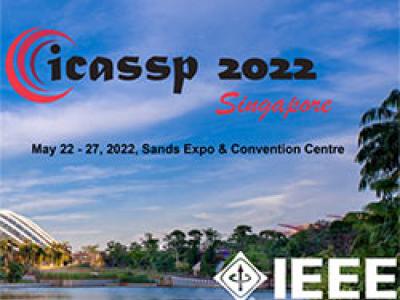
- Citation Author(s):
- Submitted by:
- Kyohei Suzuki
- Last updated:
- 5 May 2022 - 9:16am
- Document Type:
- Presentation Slides
- Document Year:
- 2022
- Event:
- Presenters:
- Kyohei Suzuki
- Paper Code:
- SPTM-8.5
- Categories:
- Keywords:
- Log in to post comments
We propose a robust approach to recovering jointly sparse signals in the presence of outliers. The robust recovery task is cast as a convex optimization problem involving a minimax concave loss function (which is weakly convex) and a strongly convex regularizer (which ensures the overall convexity). The use of the nonconvex loss makes the problem difficult to solve directly by the convex optimization methods even with the well-established firm shrinkage. We circumvent this difficulty by reformulating the problem via the Moreau decomposition so that the objective function becomes a sum of convex functions that can be minimized by the primal-dual splitting method. The parameter designs/ranges for the present specific case are derived to ensure the convergence. We demonstrate the remarkable robustness of the proposed approach against outliers by extensive simulations to the application of multi-lead electrocardiogram as well as synthetic data.