Documents
Poster
UNSUPERVISED DEEP LEARNING NETWORK FOR DEFORMABLE FUNDUS IMAGE REGISTRATION
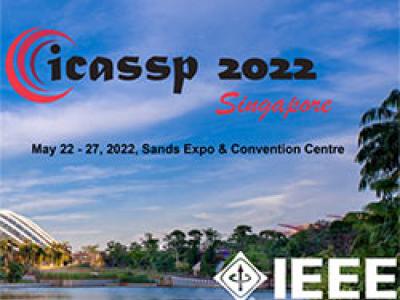
- Citation Author(s):
- Submitted by:
- Giovana Augusta...
- Last updated:
- 5 May 2022 - 11:32am
- Document Type:
- Poster
- Document Year:
- 2022
- Event:
- Presenters:
- Giovana Augusta Benvenuto
- Paper Code:
- 4973
- Categories:
- Log in to post comments
In ophthalmology and vision science applications, the process of registering a pair of fundus images, captured at different scales and viewing angles, is of paramount importance to support the diagnosis of diseases and routine eye examinations. Aiming at addressing the retina registration problem from the Deep Learning perspective, in this paper we introduce an end-to-end framework capable of learning the registration task in a fully unsupervised way. The designed approach combines Convolutional Neural Networks and Spatial Transformation Network into a unified pipeline that takes a similarity metric to gauge the difference between the images, thus enabling the image alignment without requiring any ground-truth data. Once the model is fully trained, it can perform one-shot registrations by just providing as input the pair of fundus images. As shown in the validation study, the trained model is able to successfully deal with several categories of fundus images, surpassing other recent techniques for retina registration.