Documents
Poster
GRAPH ATTENTIVE FEATURE AGGREGATION FOR TEXT-INDEPENDENT SPEAKER VERIFICATION
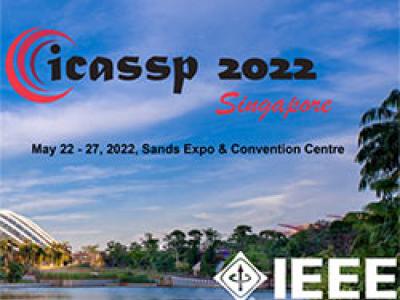
- Citation Author(s):
- Submitted by:
- Jungwoo Heo
- Last updated:
- 6 May 2022 - 2:55am
- Document Type:
- Poster
- Document Year:
- 2022
- Event:
- Presenters:
- Jungwoo Heo
- Paper Code:
- SPE-68.4
- Categories:
- Log in to post comments
The objective of this paper is to combine multiple frame-level features into a single utterance-level representation considering pair wise relationships. For this purpose, we propose a novel graph attentive feature aggregation module by interpreting each frame-level feature as a node of a graph. The inter-relationship between all possible pairs of features, typically exploited indirectly, can be directly modeled using a graph. The module comprises a graph attention layer and a graph pooling layer followed by a readout operation. The graph attention layer first models the non-Euclidean data manifold between different nodes. Then, the graph pooling layer discards less informative nodes considering the significance of the nodes. Finally, the readout operation combines the remaining nodes into a single representation. We employ two recent systems, SEResNet and RawNet2, with different input features and architectures and demonstrate that the proposed feature aggregation module consistently shows a relative improvement over 10%, compared to the baseline.