Documents
Presentation Slides
A Variational Bayesian Approach to Learning Latent Variables for Acoustic Knowledge Transfer
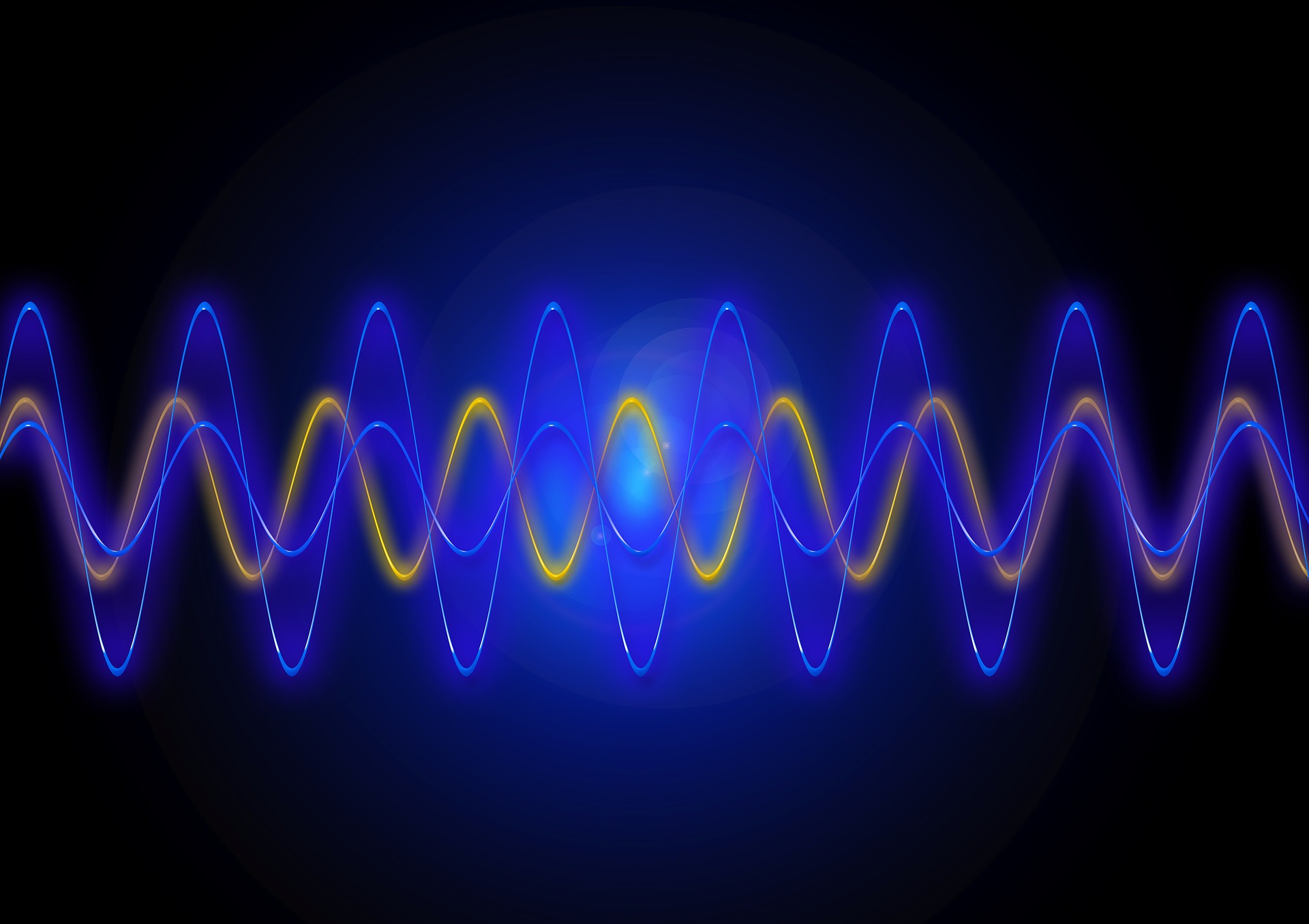
- Citation Author(s):
- Submitted by:
- Hu Hu
- Last updated:
- 6 May 2022 - 1:43am
- Document Type:
- Presentation Slides
- Document Year:
- 2022
- Categories:
- Log in to post comments
We propose a variational Bayesian (VB) approach to learning distributions of latent variables in deep neural network (DNN) models for cross-domain knowledge transfer, to address acoustic mismatches between training and testing conditions. Instead of carrying out point estimation in conventional maximum a posteriori estimation with a risk of having a curse of dimensionality in estimating a huge number of model parameters, we focus our attention on estimating a manageable number of latent variables of DNNs via a VB inference framework. To accomplish model transfer, knowledge learnt from a source domain is encoded in prior distributions of latent variables and optimally combined, in a Bayesian sense, with a small set of adaptation data from a target domain to approximate the corresponding posterior distributions. Experimental results on device adaptation in acoustic scene classification show that our proposed VB approach can obtain good improvements on target devices, and consistently outperforms 13 state-of-the-art knowledge transfer algorithms.