Documents
Poster
BNU: A BALANCE-NORMALIZATION-UNCERTAINTY MODEL FOR INCREMENTAL EVENT DETECTION
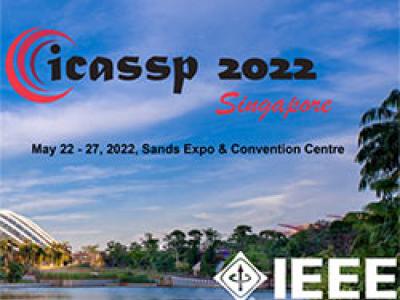
- Citation Author(s):
- Submitted by:
- Jia Li
- Last updated:
- 7 May 2022 - 12:13pm
- Document Type:
- Poster
- Document Year:
- 2022
- Event:
- Presenters:
- Jia Li
- Paper Code:
- SU3.E.4
- Categories:
- Keywords:
- Log in to post comments
Event detection is challenging in real-world application since new events continually occur and old events still exist which may result in repeated labeling for old events. There- fore, incremental event detection is essential where a model continuously learns new events and meanwhile prevents per- formance from degrading on old events. Although existing incremental event detection models achieve impressive per- formance, they face the data imbalance problem between old classes and new classes, and have the knowledge transfer prob- lem which cannot adequately utilize the knowledge provided by the previous model and data. To this end, we propose a Balance-Normalization-Uncertainty (BNU) model to address above problems. Specifically, in order to mitigate the adverse effects of data imbalance, we incorporate a balanced fine- tuning stage and a cosine normalization module. Meanwhile, we consider aleatoric uncertainty to preserve previous knowl- edge while training for new events. Experimental results show that our proposed method resolves the above challenges effec- tively and achieves consistent and significant performance on ACE and TAC KBP datasets.