Documents
Presentation Slides
Presentation Slides
Neural Collapse in Deep Homogeneous Classifiers and the Role of Weight Decay
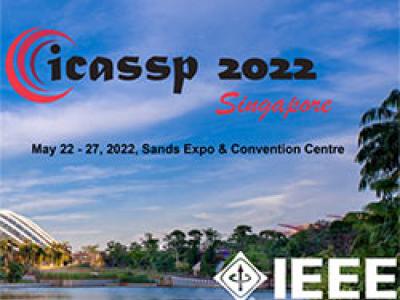
- Citation Author(s):
- Submitted by:
- Akshay Rangamani
- Last updated:
- 12 May 2022 - 9:10am
- Document Type:
- Presentation Slides
- Document Year:
- 2022
- Event:
- Presenters:
- Akshay Rangamani
- Paper Code:
- MLSP-41.4
- Categories:
- Log in to post comments
Neural Collapse is a phenomenon recently discovered in deep classifiers where the last layer activations collapse onto their class means, while the means and last layer weights take on the structure of dual equiangular tight frames. In this paper we present results showing the role of weight decay in the emergence of Neural Collapse in deep homogeneous networks. We show that certain near-interpolating minima of deep networks satisfy the Neural Collapse condition, and this can be derived from the gradient flow on the regularized square loss. We also show that weight decay is necessary for neural collapse to occur. We support our theoretical analysis with experiments that confirm our results.