Documents
Poster
Point Cloud Geometry Compression via Density-Constrained Adaptive Graph Convolution
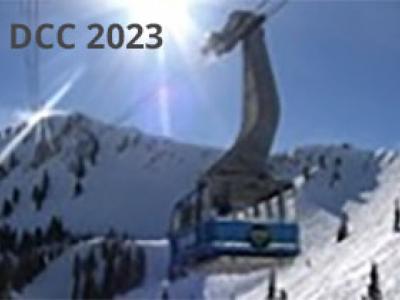
- Citation Author(s):
- Submitted by:
- Dan Wang
- Last updated:
- 13 February 2023 - 1:25am
- Document Type:
- Poster
- Document Year:
- 2023
- Event:
- Presenters:
- Dan Wang
- Paper Code:
- DCC-222
- Categories:
- Log in to post comments
With the rapid development of 3D vision applications such as autonomous driving and the dramatic increase of point cloud data, it becomes critical to efficiently compress 3D point clouds. Recently, point-based point cloud compression has attracted great attention due to its superior performance at low bit rates. However, lacking an efficient way to represent the local geometric correlation well, most existing methods can hardly extract fine local features accurately. Thus it’s difficult for them to obtain high-quality reconstruction of local geometry of point clouds. To solve this problem, we design a density-constrained adaptive graph convolution (DCAGC) to efficiently ensure the consistency of local geometry between original and reconstructed point clouds. Furthermore, we propose a novel point cloud compression method based on DCAGC under variational autoencoder (VAE) framework, in which the point clouds are hierarchically reconstructed and gradually refined. Experimental results demonstrate that our method outperforms the state-of-the-arts in terms of rate-distortion performance with average 47% D1 BD Bitrate (BDBR) and 45% D2 BDBR gain, and achieves more satisfactory reconstructions with clearer geometric details.