Documents
Presentation Slides
A Contrastive Knowledge Transfer Framework for Model Compression and Transfer Learning
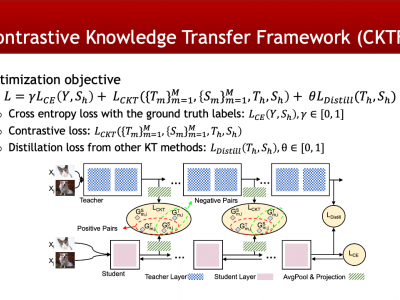
- Citation Author(s):
- Submitted by:
- Kaiqi Zhao
- Last updated:
- 19 May 2023 - 7:21pm
- Document Type:
- Presentation Slides
- Document Year:
- 2023
- Event:
- Presenters:
- Kaiqi Zhao
- Paper Code:
- https://github.com/kaiqi123/CKTF.git
- Categories:
- Keywords:
- Log in to post comments
Knowledge Transfer (KT) achieves competitive performance and is widely used for image classification tasks in model compression and transfer learning. Existing KT works transfer the information from a large model ("teacher") to train a small model ("student") by minimizing the difference of their conditionally independent output distributions. However, these works overlook the high-dimension structural knowledge from the intermediate representations of the teacher, which leads to limited effectiveness, and they are motivated by various heuristic intuitions, which makes it difficult to generalize. This paper proposes a novel Contrastive Knowledge Transfer Framework (CKTF), which enables the transfer of sufficient structural knowledge from the teacher to the student by optimizing multiple contrastive objectives across the intermediate representations between them. Also, CKTF provides a generalized agreement to existing KT techniques and increases their performance significantly by deriving them as specific cases of CKTF. The extensive evaluation shows that CKTF consistently outperforms the existing KT works by 0.04% to 11.59% in model compression and by 0.4% to 4.75% in transfer learning on various models and datasets.
Comments
Submit the code, slides, and
Submit the code, slides, and video of our paper