Documents
Presentation Slides
A study on the invariance in security whatever the dimension of images for the steganalysis by deep-learning
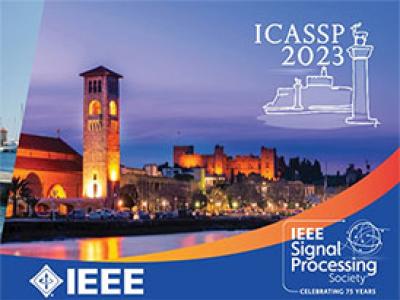
- Citation Author(s):
- Submitted by:
- Marc CHAUMONT
- Last updated:
- 31 May 2023 - 4:01pm
- Document Type:
- Presentation Slides
- Document Year:
- 2023
- Event:
- Presenters:
- CHAUMONT
- Paper Code:
- Paper n° 906 IFS-P4: Watermarking, Data Hiding and Human Factors in Security
- Categories:
- Log in to post comments
In this paper, we study the performance invariance of convolutional neural networks when confronted with variable image sizes in the context of a more ”wild steganalysis”. First, we propose two algorithms and definitions for a fine experimental protocol with datasets owning ”similar difficulty” and ”similar security”. The ”smart crop 2” algorithm allows the introduction of the Nearly Nested Image Datasets (NNID) that ensure ”a similar difficulty” between various datasets, and a dichotomous research algorithm allows a ”similar security”. Second, we show that invariance does not exist in state-of-the-art architectures. We also exhibit a difference in behavior depending on whether we test on images larger or smaller than the training images. Finally, based on the experiments, we propose to use the dilated convolution which leads to an improvement of a state-of-the-art architecture