Documents
Poster
Poster
Multi-dimensional Signal Recovery Using Low-rank Deconvolution
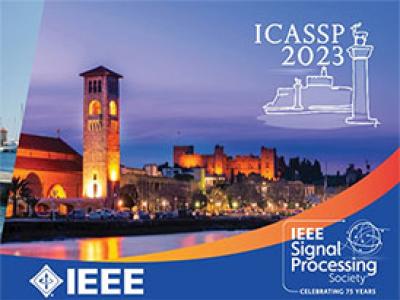
- DOI:
- 10.60864/hmpj-sd38
- Citation Author(s):
- Submitted by:
- David Reixach
- Last updated:
- 17 November 2023 - 12:07pm
- Document Type:
- Poster
- Document Year:
- 2023
- Event:
- Presenters:
- David Reixach
- Categories:
- Log in to post comments
In this work we present Low-rank Deconvolution, a powerful framework for low-level feature-map learning for efficient signal representation with application to signal recovery. Its formulation in multi-linear algebra inherits properties from convolutional sparse coding and low-rank approximation methods as in this setting signals are decomposed in a set of filters convolved with a set of low-rank tensors. We show its advantages by learning compressed video representations and solving image in-painting problems.