Documents
Research Manuscript
Building Blocks for a Complex-Valued Transformer Architecture
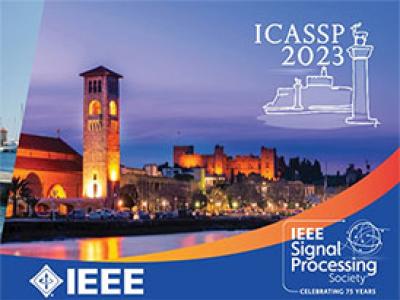
- DOI:
- 10.60864/r393-et27
- Citation Author(s):
- Submitted by:
- Florian Eilers
- Last updated:
- 17 November 2023 - 12:07pm
- Document Type:
- Research Manuscript
- Document Year:
- 2023
- Event:
- Presenters:
- Florian Eilers
- Paper Code:
- (MLSP-P17.10
- Categories:
- Log in to post comments
Most deep learning pipelines are built on real-valued operations to deal with real-valued inputs such as images, speech or music signals. However, a lot of applications naturally make use of complex-valued signals or images, such as MRI or remote sensing. Additionally the Fourier transform of signals is complex-valued and has numerous applications. We aim to make deep learning directly applicable to these complex-valued signals without using projections into R2 . Thus we add to the recent developments of complex-valued neural networks by presenting building blocks to transfer the transformer architecture to the complex domain. We present multiple versions of a complex-valued Scaled Dot-Product Attention mechanism as well as a complex-valued layer normalization. We test on a classification and a sequence generation task on the MusicNet dataset and show improved robustness to overfitting while maintaining on-par performance when compared to the real-valued transformer architecture.