Documents
Poster
SEMANTIC-EMBEDDED KNOWLEDGE ACQUISITION AND REASONING FOR IMAGE SEGMENTATION
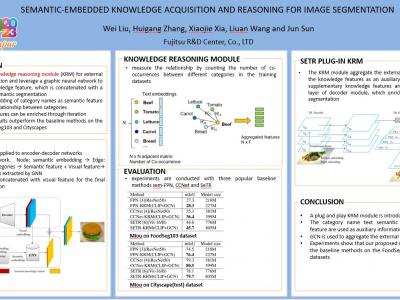
- DOI:
- 10.60864/tj0c-3g75
- Citation Author(s):
- Submitted by:
- WEI LIU
- Last updated:
- 17 November 2023 - 12:05pm
- Document Type:
- Poster
- Document Year:
- 2023
- Event:
- Presenters:
- Wei Liu
- Categories:
- Log in to post comments
Image segmentation is a difficult and challenging task because of the complex object appearance and diverse object categories. Traditional methods directly use visual features for segmentation but ignore the correlation between objects. We introduce a knowledge reasoning module (KRM) for external knowledge aggregation and leverage a graphic neural network to aggregate the knowledge feature, which is concatenated with a visual feature for semantic segmentation. To this end, we use word embedding of category names as semantic feature and establish the relationship between categories. Through iteration, the aggregated features can be enriched. In experiments, three well known semantic segmentation methods are used as baseline. Our experiment results outperform the baseline methods on the food dataset FoodSeg103 and Cityscapes, and demonstrate the effectiveness of our proposed method.