Documents
Poster
Deep Versatile Hyperspectral Reconstruction Model from a Snapshot Measurement with Arbitrary Masks
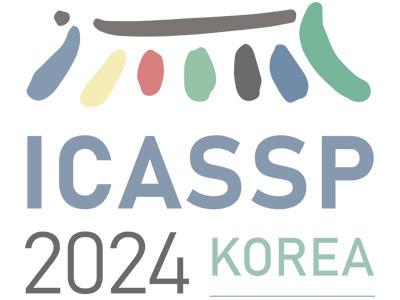
- DOI:
- 10.60864/r4b9-pd65
- Citation Author(s):
- Submitted by:
- Xian-Hua Han
- Last updated:
- 6 June 2024 - 10:50am
- Document Type:
- Poster
- Document Year:
- 2024
- Event:
- Presenters:
- Xian-Hua Han
- Paper Code:
- CI-P1.2
- Categories:
- Log in to post comments
Recently, coded aperture snapshot spectral imaging (CASSI) has been actively researched to capture three-dimensional (3D) hyperspectral (HS) images for dynamic scenes, where the optical systems detect a 2D snapshot measurement while a computational algorithm performs the inverse problem for recovering the latent HS cubic data. Benefiting from the powerful modeling capability of the deep convolution neural networks (DCNN), the reconstruction performance of the HS images has been significantly improved. However, the existing deep methods usually assume a particular hardware mask to train the reconstruction models, and restrict widely applicability to the snapshots measured in different hardwares. This study exploits a novel deep versatile HS reconstruction framework for adaptively handling the snapshots with arbitrary masks. Specifically, we employ a meta-learning like training procedure using the training paired samples of different distributions to learn a highly generalized model, and further incorporate a mask structure modeling module to produce effective knowledge for modulating the spectral recovering procedure. Moreover, we configure the deep reconstruction model with the spectral transformer for modeling the long-dependence in spectral domain, which is especially critical for high fidelity spectral recovering. Experiments on two benchmark HS datasets have demonstrated the superiority of our framework over the state-of-the-art methods.
ID1717.pdf

Comments
None
None