Documents
Presentation Slides
Presentation Slides
Unravel Anomalies: An End-to-end Seasonal-Trend Decomposition Approach for Time Series Anomaly Detection
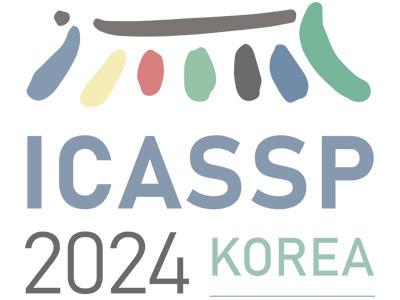
- DOI:
- 10.60864/3hsc-x603
- Citation Author(s):
- Submitted by:
- Zhenwei Zhang
- Last updated:
- 6 June 2024 - 10:27am
- Document Type:
- Presentation Slides
- Document Year:
- 2024
- Event:
- Presenters:
- Zhenwei Zhang
- Paper Code:
- https://github.com/zhangzw16/TADNet
- Categories:
- Log in to post comments
Traditional Time-series Anomaly Detection (TAD) methods often struggle with the composite nature of complex time-series data and a diverse array of anomalies. We introduce TADNet, an end-to-end TAD model that leverages Seasonal-Trend Decomposition to link various types of anomalies to specific decomposition components, thereby simplifying the analysis of complex time-series and enhancing detection performance. Our training methodology, which includes pre-training on a synthetic dataset followed by fine-tuning, strikes a balance between effective decomposition and precise anomaly detection. Experimental validation on real-world datasets confirms TADNet’s state-of-the-art performance across a diverse range of anomalies.
Links: