Documents
Presentation Slides
Learning Graphs and Simplicial Complexes from Data
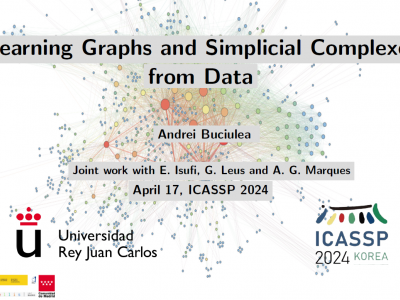
- DOI:
- 10.60864/1x1b-0085
- Citation Author(s):
- Submitted by:
- Andrei Buciulea
- Last updated:
- 6 June 2024 - 10:23am
- Document Type:
- Presentation Slides
- Document Year:
- 2024
- Event:
- Presenters:
- Andrei Buciulea Vlas
- Paper Code:
- SPTM-L3.6
- Categories:
- Keywords:
- Log in to post comments
Graphs are widely used to represent complex information and signal domains with irregular support. Typically, the underlying graph topology is unknown and must be estimated from the available data. Common approaches assume pairwise node interactions and infer the graph topology based on this premise. In contrast, our novel method not only unveils the graph topology but also identifies three-node interactions, referred to in the literature as second-order simplicial complexes (SCs). We model signals using a graph autoregressive Volterra framework, enhancing it with structured graph Volterra kernels to learn SCs. We propose a mathematical formulation for graph and SC inference, solving it through convex optimization involving group norms and mask matrices. Experimental results on synthetic and real-world data showcase a superior performance for our approach compared to existing methods.