Documents
Poster
SGT: SELF-GUIDED TRANSFORMER FOR FEW-SHOT SEMANTIC SEGMENTATION
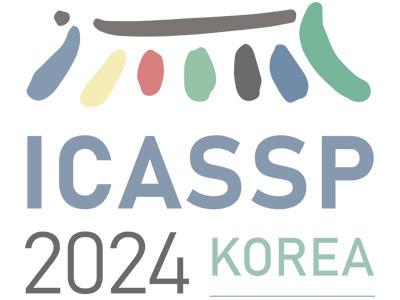
- DOI:
- 10.60864/pjms-jh07
- Citation Author(s):
- Submitted by:
- k ai
- Last updated:
- 6 June 2024 - 10:23am
- Document Type:
- Poster
- Document Year:
- 2024
- Event:
- Paper Code:
- MLSP-P13
- Categories:
- Log in to post comments
For the few-shot segmentation (FSS) task, existing methods
attempt to capture the diversity of new classes by fully uti-
lizing the limited support images, such as cross-attention and
prototype matching. However, they often overlook the fact
that there is variability in different regions of the same ob-
ject, and intra-image similarity is higher than inter-image sim-
ilarity.To address these limitations, a Self-Guided Trans-
former (SGT) is proposed by leveraging intra-image similar-
ity to improve intra-object inconsistencies in this paper. The
proposed SGT can selectively guide segmentation, emphasiz-
ing the regions that are easily distinguishable while adapting
to the challenges caused by less discriminative regions within
objects. Through a refined feature interaction scheme and the
novel SGT module, our method can achieve state-of-the-art
performance on various FSS datasets, demonstrating signifi-
cant advances in few-shot semantic segmentation. The code
is publicly available at https://github.com/HuHaigen/SGT.