Documents
Poster
ENHANCING NOISY LABEL LEARNING VIA UNSUPERVISED CONTRASTIVE LOSS WITH LABEL CORRECTION BASED ON PRIOR KNOWLEDGE
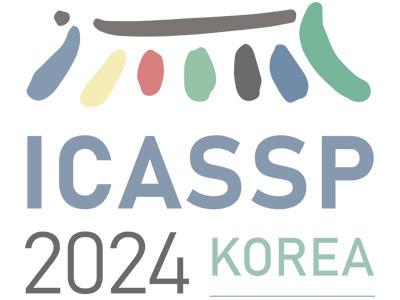
- DOI:
- 10.60864/ntb4-d394
- Citation Author(s):
- Submitted by:
- Masaki Kashiwagi
- Last updated:
- 6 June 2024 - 10:21am
- Document Type:
- Poster
- Document Year:
- 2024
- Event:
- Presenters:
- Masaki Kashiwagi
- Paper Code:
- MLSP-P1.6
- Categories:
- Keywords:
- Log in to post comments
To alleviate the negative impacts of noisy labels, most of the noisy label learning (NLL) methods dynamically divide the training data into two types, “clean samples” and “noisy samples”, in the training process. However, the conventional selection of clean samples heavily depends on the features learned in the early stages of training, making it difficult to guarantee the cleanliness of the selected samples in scenarios where the noise ratio is high. In addition, their optimization processes are based on the supervised loss including noisy labels, and effective representation cannot be obtained in the presence of a large number of noisy labels. To address these problems, we propose an effective method capable of robustly performing NLL under extremely high noise ratios.
In the proposed method, by introducing the prior knowledge of a pre-trained vision and language model, we can effectively select clean samples since it does not depend on the learning process of NLL. Moreover, the introduction of an unsupervised contrastive learning approach enables the acquisition of noise-robust feature representations in SSL. Experiments with synthetic label noise on CIFAR-10 and CIFAR-100, the benchmark datasets in NLL, demonstrate that the proposed method significantly outperforms state-of-the-art methods.