Documents
Poster
Giraffe: A Genetic Programming Algorithm To Build Deep Learning Ensembles For Ecg Arrhythmia Classification
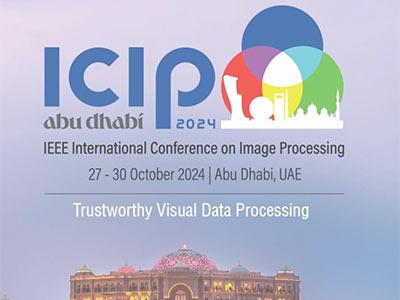
- Citation Author(s):
- Submitted by:
- Jakub Nalepa
- Last updated:
- 10 November 2024 - 6:47pm
- Document Type:
- Poster
- Document Year:
- 2024
- Event:
- Presenters:
- Damian Kucharski
- Categories:
- Log in to post comments
Cardiovascular diseases remain one of the leading causes of death worldwide. Therefore, developing and validating automated tools to help identify high-risk patients are of paramount clinical utility. In this article, we tackle this task and introduce a genetic programming algorithm (called GIRAFFE) to build (deep) machine learning classification ensembles for arrhythmia classification from two-dimensional images of 12-lead electrocardiogram (ECG) tracings. GIRAFFE evolves the architecture, content, and fusion scheme of the ensemble, to obtain an accurate yet lightweight classification system. The experimental study performed over a large-scale dataset of ECG images revealed that our approach outperforms other ensemble methods and carefully fine-tuned deep models, elaborates compact heterogeneous ensembles, and does not require any user intervention hence it is easy to apply to other classification tasks.