Documents
Poster
Accurate colon segmentation using 2D convolutional neural networks with 3D contextual information
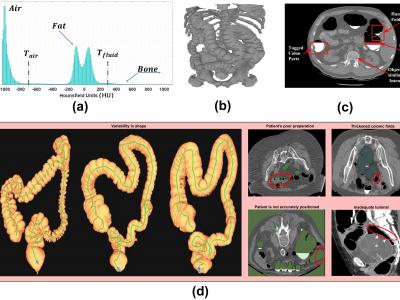
- DOI:
- 10.60864/0edr-as87
- Citation Author(s):
- Submitted by:
- Samir Harb
- Last updated:
- 12 November 2024 - 10:07pm
- Document Type:
- Poster
- Document Year:
- 2024
- Event:
- Presenters:
- Samir Farag Harb
- Paper Code:
- MP2.PB.11
- Categories:
- Keywords:
- Log in to post comments
This study introduces an innovative framework for accurate colon segmentation in abdomen CT scans, addressing the unique challenges of this task. Our architecture enhances well-established 2D segmentation models by incorporating 3D contextual information through a novel method that generates an attention map for a given slice by considering its neighboring slices. This approach achieves effective colon segmentation without complex 3D convolutional neural networks (CNNs) or Long Short-Term Memory (LSTM) networks by combining 2D CNNs. Validated on a dataset of 98 CT scans from 49 patients, the architecture demonstrates notable performance, capturing crucial details for precise colon segmentation. The experiments include thorough model selection and cross-validation, providing insights into the efficacy of our approach. The results highlight the potential for streamlined colon segmentation in medical imaging by integrating 2D and 3D information with 2D networks, avoiding challenges associated with 3D networks. The code for the model architecture is available at: https://github.com/Samir-Farag/ICIP2024.git.