Documents
Supplementary Materials
ICIP2025_Supplementary_ESCANet
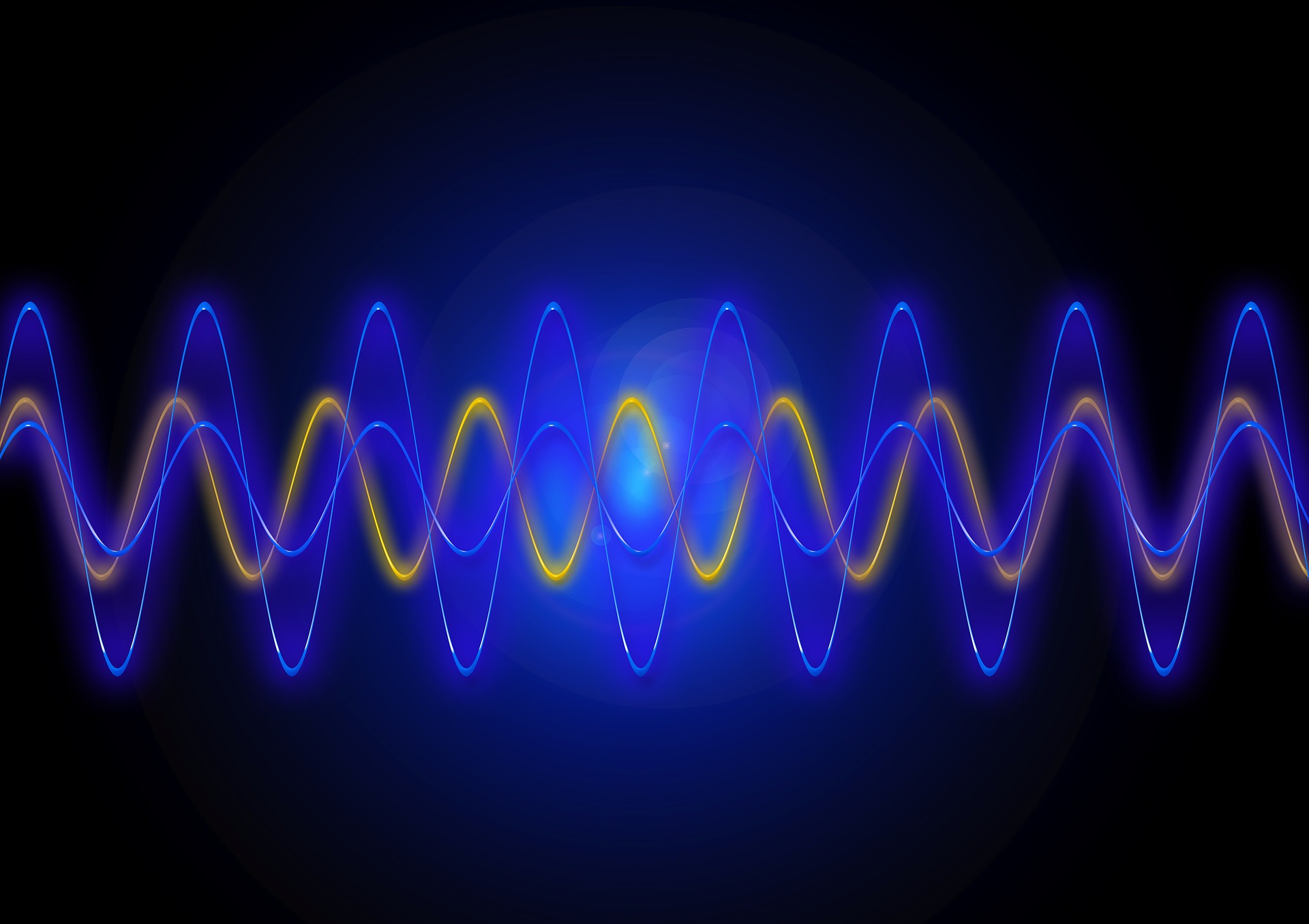
- Citation Author(s):
- Submitted by:
- Donggeun Ko
- Last updated:
- 13 January 2025 - 12:31am
- Document Type:
- Supplementary Materials
- Document Year:
- 2025
- Presenters:
- Donggeun Ko
- Paper Code:
- 1046
- Categories:
- Keywords:
- Log in to post comments
While deep learning based solutions, including CNNs or transformer-based architectures, have demonstrated promising results for image super-resolution (SR) tasks, their substantial depth and parameters challenge deployment on edge computing AI-enabled devices. To address this issue, we propose a lightweight single image super-resolution (SISR) model named Efficient Spatial and Channel Attentive Network (ESCANet), comprised of Spatial Enhancement Module (SEM) and Channel-wise Enhancement Module (CEM). SEM efficiently captures both local and non-local information by introducing Efficient Self-Attention Approximation Block (ESAB) that does not increase the computational complexity. CEM modulates the local features by channel mixing. Experiments demonstrate that ESCANet achieves a reasonable trade-off between computational efficiency and reconstruction performance on five well-known SR benchmark datasets.