Documents
Research Manuscript
DEEP UNSUPERVISED DESPECKLING WITH UNBIASED RISK ESTIMATION
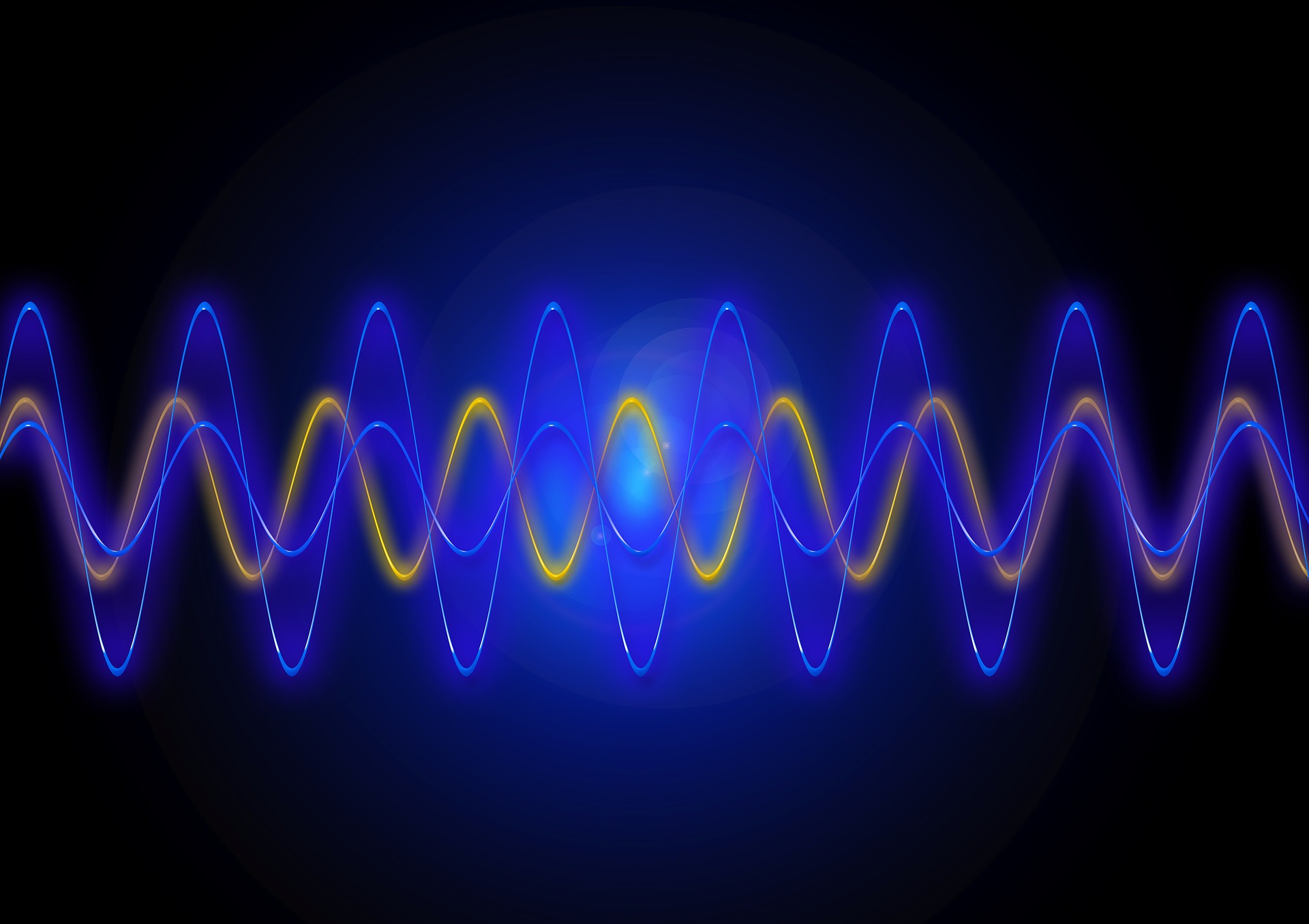
- Citation Author(s):
- Submitted by:
- Anonymous User
- Last updated:
- 3 February 2025 - 12:14pm
- Document Type:
- Research Manuscript
- Categories:
- Log in to post comments
Despeckling of Synthetic Aperture Radar (SAR) images has seen significant progress in recent years, largely driven by advancements in deep learning techniques. However, many of these approaches face challenges when applied to new SAR datasets, primarily due to their dependence on ground truth images, which are often unavailable for real-world sensors. In this paper, we address this limitation by extending the concept of unbiased risk estimation in the presence of Gamma-distributed multiplicative speckle. Specifically, we demonstrate that it is possible to train deep denoising networks without relying on ground truth data using our estimator. We introduce a new formulation of the Multiplicative Unbiased Risk Estimator (MURE) and present a computationally efficient Monte Carlo-based method that enables accurate estimation of the modified MURE cost, facilitating effective unsupervised training of deep neural networks from large datasets consisting solely of noisy SAR images. Experimental results on both synthetic datasets and real Sentinel-1 SAR images validate the suitability of our method for real-world applications. Even without ground truth, our method achieves performance that closely matches the Oracle-based denoiser and proves superior to the out-of-domain performance of popular supervised SAR despeckling methods. This supplementary material contains proof of some key results in the main paper.