Documents
Poster
Feature Mapping, Score-, and Feature-Level Fusion for Improved Normal and Whispered Speech Speaker Verification
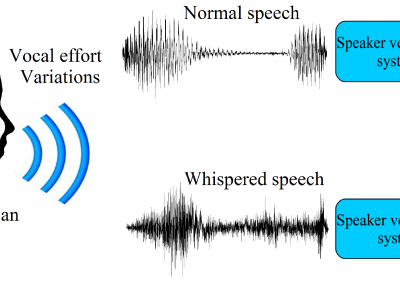
- Citation Author(s):
- Submitted by:
- Milton Sarria-Paja
- Last updated:
- 19 March 2016 - 6:18pm
- Document Type:
- Poster
- Document Year:
- 2016
- Event:
- Presenters:
- Milton Sarria-Paja
- Categories:
- Log in to post comments
In this paper, automatic speaker verification using normal and whispered speech is explored. Typically, for speaker verification systems with varying vocal effort inputs, standard solutions such as feature mapping or addition of data during parameter estimation (training) and enrollment stages result in a trade-off between accuracy gains with whispered test data and accuracy losses (up to 70% in equal error rate, EER) with normal test data. To overcome this shortcoming, this paper proposes two innovations. First, we show the complementarity of features derived from AM-FM models over conventional mel-frequency cepstral coefficients, thus signalling the importance of instantaneous phase information for whispered speech speaker verification. Next, two fusion schemes are explored: score- and feature-level fusion. Overall, we show that gains as high as 30% and 84% in EER can be achieved for normal and whispered speech, respectively, using feature-level fusion.
ICASSP2016.pdf
