Documents
Presentation Slides
Alleviating Hallucinations via Supportive Window Indexing in Abstractive Summarization
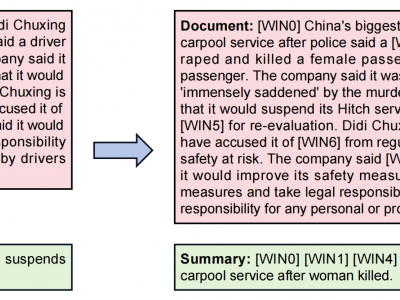
- Citation Author(s):
- Submitted by:
- Jiaxin Duan
- Last updated:
- 10 April 2024 - 12:27pm
- Document Type:
- Presentation Slides
- Document Year:
- 2024
- Event:
- Presenters:
- Jiaxin Duan
- Paper Code:
- 5296
- Categories:
- Keywords:
- Log in to post comments
Abstractive summarization models learned with maximum likelihood estimation (MLE) have been proven to produce hallucinatory content, which heavily limits their real-world
applicability. Preceding studies attribute this problem to the semantic insensitivity of MLE, and they compensate for it with additional unsupervised learning objectives that maximize the metrics of document-summary inferring, however, resulting in unstable and expensive model training. In this paper, we propose a novel supportive windows indexing
grounded summarization (SWIGS) paradigm, where an input document is split into several windows, and a summarization model orderly generates the indices of supportive windows before each summary sentence. Because the supportive windows locate the source information closely related to the summary sentence to be generated, pointing out their indices at first helps to ground the evidence-based summary generation, thus alleviating groundless hallucinations. We create only supervised objectives to learn the SWIGS model and conduct extensive experiments on two well-known datasets to validate its effectiveness. Results vouch for the superiority of SWIGS as it outperforms previous methods regarding multiple metrics.
Comments
Comment from the Review Committee
This submission proposes a novel method to reduce hallucinations in abstractive summarization tasks. Overall, the paper is well-written, and the authors conduct extensive experiments on two representative datasets. In the updated version, please provide the results from hybrid and extractive results for comprehensive comparisons following the reviewers’ suggestions and add descriptions about the SOTA methods.