Documents
Poster
Phonotactic Language Recognition using a Universal Phoneme Recognizer and a Transformer Architecture
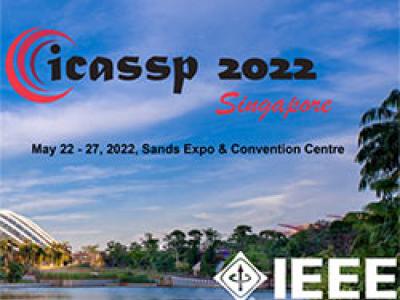
- Citation Author(s):
- Submitted by:
- David Romero
- Last updated:
- 6 May 2022 - 1:05pm
- Document Type:
- Poster
- Document Year:
- 2022
- Event:
- Presenters:
- David Romero
- Paper Code:
- 5307
- Categories:
- Keywords:
- Log in to post comments
In this paper, we describe a phonotactic language recognition model that effectively manages long and short n-gram input sequences to learn contextual phonotacticbased vector embeddings. Our approach uses a transformerbased encoder that integrates a sliding window attention to attempt finding discriminative short and long cooccurrences of language dependent n-gram phonetic units. We then evaluate and compare the use of different phoneme recognizers (Brno and Allosaurus) and sub-unit tokenizers to help select the more discriminative n-grams. The proposed architecture is evaluated using the Kalaka-3 database that contains clean and noisy audio recordings for very similar languages (i.e. Iberian languages, e.g., Spanish, Galician, Catalan). We provide results using the Cavg and accuracy metrics used in NIST evaluations. The experimental results show that our proposed approach outperforms by 21% of relative improvement to the best system presented in the Albayzin LR competition.
Poster.pdf

Comments
Paper Icassp
Paper Icassp