Documents
Presentation Slides
CAPITALIZATION NORMALIZATION FOR LANGUAGE MODELING WITH AN ACCURATE AND EFFICIENT HIERARCHICAL RNN MODEL
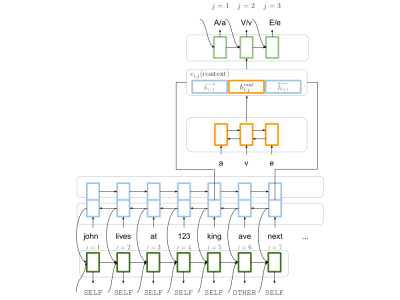
- Citation Author(s):
- Submitted by:
- Hao Zhang
- Last updated:
- 6 May 2022 - 11:38am
- Document Type:
- Presentation Slides
- Document Year:
- 2022
- Event:
- Presenters:
- Hao Zhang
- Paper Code:
- SPE-4.1
- Categories:
- Log in to post comments
Capitalization normalization (truecasing) is the task of restoring the correct case (uppercase or lowercase) of noisy text. We propose a fast, accurate and compact two-level hierarchical word-and-character-based recurrent neural network model. We use the truecaser to normalize user-generated text in a Federated Learning framework for language modeling. A case-aware language model trained on this normalized text achieves the same perplexity as a model trained on text with gold capitalization. In a real user A/B experiment, we demonstrate that the improvement translates to reduced prediction error rates in a virtual keyboard application. Similarly, in an ASR language model fusion experiment, we show reduction in uppercase character error rate and word error rate.