Documents
Poster
Computing an Entire Solution Path of a Nonconvexly Regularized Convex Sparse Model
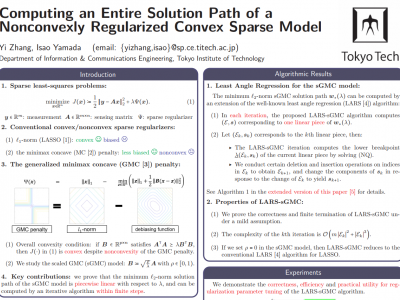
- DOI:
- 10.60864/47q6-2850
- Citation Author(s):
- Submitted by:
- Yi Zhang
- Last updated:
- 6 June 2024 - 10:21am
- Document Type:
- Poster
- Document Year:
- 2024
- Event:
- Presenters:
- Yi Zhang
- Paper Code:
- SPTM-P1.2
- Categories:
- Keywords:
- Log in to post comments
The generalized minimax concave (GMC) penalty is a nonconvex sparse regularizer which can preserve the overall-convexity of the sparse least squares problem. In this paper, we study the solution path of a special but important instance of the GMC model termed the scaled GMC (sGMC) model. We show that despite the nonconvexity of the regularizer, there exists a solution path of the sGMC model which is piecewise linear as a function of the regularization parameter, and we propose an efficient algorithm for computing a solution path of this type. Our algorithm is an extension of the well-known least angle regression (LARS) algorithm for LASSO, hence we term the proposed algorithm LARS-sGMC. The proposed algorithm is provably correct and finitely terminating under suitable assumptions. Numerical experiments verify the correctness of LARS-sGMC, and demonstrate the usefulness of LARS-sGMC (with proper model selection criterion) for finding the optimal regularization parameter of the sGMC model.