Documents
Poster
Deep Learning for Classroom Activity Detection from Audio
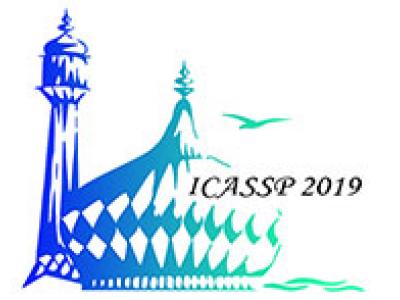
- Citation Author(s):
- Submitted by:
- Robin Cosbey
- Last updated:
- 10 May 2019 - 4:33pm
- Document Type:
- Poster
- Document Year:
- 2019
- Event:
- Paper Code:
- MLSP-P15
- Categories:
- Log in to post comments
Increasingly, post-secondary instructors are incorporating innovative teaching practices into their classrooms to improve student learning outcomes. In order to assess the effect of these techniques, it is helpful to quantify the types of activity being conducted in the classroom. Unfortunately, self-reporting is unreliable and manual annotation is tedious and scales poorly. We introduce a set of deep learning classifiers for automatic activity annotation, evaluating them on a collection of classroom recordings, with frames labeled as “single-voice” (primarily lecture), “multi-voice” (primarily group discussion), “no-voice” (primarily silent work) or “other.” We
find that our best approach obtains a 7.1% frame error rate (92.7% weighted F-measure) on held out class sessions from previously seen instructors (a 7 hr test set), and 10.1% error (89.1% weighted F-measure) on previously unseen instructors (a separate 18 hr test set). These represent 32% and 45% relative reductions in error over the existing state-of-the-art for this task. We also show that our estimates of how much classroom time spent per task are better correlated with actual time spent than existing systems.