Documents
Presentation Slides
An Efficient Active Set Algorithm for Covariance Based Joint Data and Activity Detection for Massive Random Access with Massive MIMO
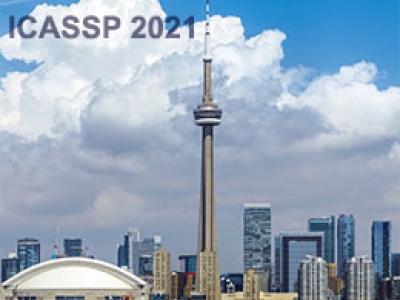
- Citation Author(s):
- Submitted by:
- Ziyue Wang
- Last updated:
- 16 June 2021 - 8:42am
- Document Type:
- Presentation Slides
- Event:
- Categories:
- Log in to post comments
This paper proposes a computationally efficient algorithm to solve the joint data and activity detection problem for massive random access with massive multiple-input multiple-output (MIMO). The BS acquires the active devices and their data by detecting the transmitted preassigned nonorthogonal signature sequences. This paper employs a covariance based approach that formulates the detection problem as a maximum likelihood estimation (MLE) problem. To efficiently solve the problem, this paper designs a novel iterative algorithm with low complexity in the regime where the device activity pattern is sparse -- a key feature that existing algorithmic designs have not previously exploited for reducing complexity. Specifically, at each iteration, the proposed algorithm focuses on only a small subset of all potential sequences, namely the active set, which contains a few most likely active sequences (i.e., transmitted sequences by all active devices), and performs the detection for the sequences in the active set. The active set is carefully selected at each iteration based on the current detection result and the first-order optimality condition of the MLE problem. Simulation results show that the proposed active set algorithm enjoys significantly better computational efficiency (in terms of the CPU time) than the state-of-the-art algorithms.