Documents
Poster
END-TO-END FEEDBACK LOSS IN SPEECH CHAIN FRAMEWORK VIA STRAIGHT-THROUGH ESTIMATOR
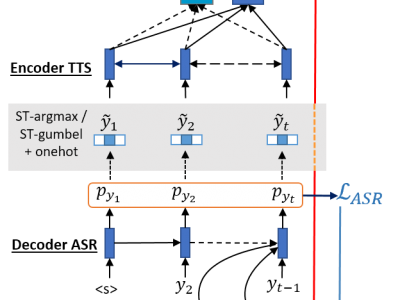
- Citation Author(s):
- Submitted by:
- Andros Tjandra
- Last updated:
- 14 May 2019 - 8:26pm
- Document Type:
- Poster
- Document Year:
- 2019
- Event:
- Presenters:
- Andros Tjandra
- Paper Code:
- SLP-P7.8
- Categories:
- Log in to post comments
The speech chain mechanism integrates automatic speech recognition (ASR) and text-to-speech synthesis (TTS) modules into a single cycle during training. In our previous work, we applied a speech chain mechanism as a semi-supervised learning. It provides the ability for ASR and TTS to assist each other when they receive unpaired data and let them infer the missing pair and optimize the model with reconstruction loss. If we only have speech without transcription, ASR generates the most likely transcription from the speech data, and then TTS uses the generated transcription to reconstruct the original speech features. However, in previous papers, we just limited our back-propagation to the closest module, which is the TTS part. One reason is that back-propagating the error through the ASR is challenging due to the output of the ASR being discrete tokens, creating non-differentiability between the TTS and ASR. In this paper, we address this problem and describe how to thoroughly train a speech chain end-to-end for reconstruction loss using a straight-through estimator (ST). Experimental results revealed that, with sampling from ST-Gumbel-Softmax, we were able to update ASR parameters and improve the ASR performances by 11\% relative CER reduction compared to the baseline.