Documents
Presentation Slides
[ICASSP 2022] NOT ALL FEATURES ARE EQUAL: SELECTION OF ROBUST FEATURES FOR SPEECH EMOTION RECOGNITION IN NOISY ENVIRONMENTS
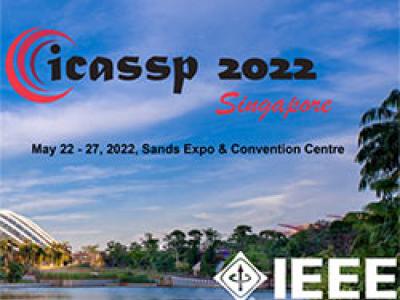
- Citation Author(s):
- Submitted by:
- Seong-Gyun Leem
- Last updated:
- 4 May 2022 - 5:09pm
- Document Type:
- Presentation Slides
- Document Year:
- 2022
- Event:
- Presenters:
- Seong-Gyun Leem
- Paper Code:
- SPE-15.5
- Categories:
- Log in to post comments
Speech emotion recognition (SER) system deployed in real-world applications often encounters noisy speech. While most noise compensation techniques consider all acoustic features to have equal impact on the SER model, some acoustic features may be more sensitive to noisy conditions. This paper investigates the noise robustness of each feature in the acoustic feature set. We focus on low-level descriptors (LLDs) commonly used in SER systems. We firstly train SER models with clean speech by only using a single LLD. Then, we rank each LLD with respect to the absolute performance on a development set contaminated with noise, and the relative performance decrease from the results from the models trained with the clean set. Our experiment shows that using all the LLDs leads to worse performance than training the system with a single robust LLD. We propose to select a group of robust features according to their performance and robustness in noisy condition. Without using any compensation method, our feature selection methods improve the performance by 24.4% (arousal), 23.9% (dominance), and 43.2% (valence) in the 10dB noisy condition. Moreover, even though the selection is conducted with the 10dB condition, our selection methods also yield performance improvements in unseen noisy recording conditions.