Documents
Poster
Infant Crying Detection in Real-World Environments
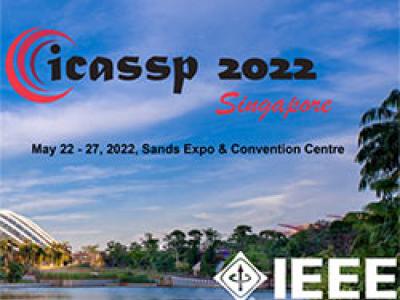
- Citation Author(s):
- Submitted by:
- Xuewen YAO
- Last updated:
- 4 May 2022 - 6:58pm
- Document Type:
- Poster
- Document Year:
- 2022
- Event:
- Presenters:
- Xuewen Yao
- Paper Code:
- AUD-2.1
- Categories:
- Log in to post comments
Most existing cry detection models have been tested with data collected in controlled settings. Thus, the extent to which they generalize to noisy and lived environments is unclear. In this paper, we evaluate several established machine learning approaches including a model leveraging both deep spectrum and acoustic features. This model was able to recognize crying events with F1 score 0.613 (Precision: 0.672, Recall: 0.552), showing improved external validity over existing methods at cry detection in everyday real-world settings. As part of our evaluation, we collect and annotate a novel dataset of infant crying compiled from over 780 hours of labeled real-world audio data, captured via recorders worn by infants in their homes, which we make publicly available. Our findings confirm that a cry detection model trained on in-lab data underperforms when presented with real-world data (in-lab test F1: 0.656, real-world test F1: 0.236), highlighting the value of our new dataset and model.