Documents
Presentation Slides
A Joint Convolutional and Spatial Quad-Directional LSTM Network for Phase Unwrapping
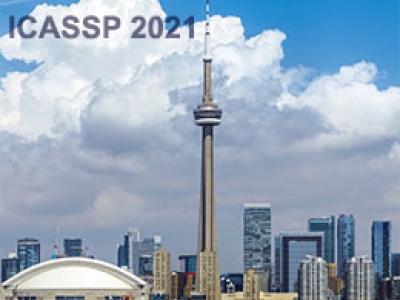
- Citation Author(s):
- Submitted by:
- Laknath de Silva
- Last updated:
- 22 June 2021 - 12:50pm
- Document Type:
- Presentation Slides
- Document Year:
- 2021
- Event:
- Presenters:
- Malsha V. Perera
- Paper Code:
- MLSP-46.4
- Categories:
- Log in to post comments
Phase unwrapping is a classical ill-posed problem which aims to recover the true phase from wrapped phase. In this paper, we introduce a novel Convolutional Neural Network (CNN) that incorporates a Spatial Quad-Directional Long Short Term Memory (SQD-LSTM) for phase unwrapping, by formulating it as a regression problem. Incorporating SQD-LSTM can circumvent the typical CNNs' inherent difficulty of learning global spatial dependencies which are vital when recovering the true phase. Furthermore, we employ a problem specific composite loss function to train this network. The proposed network is found to be performing better than the existing methods under severe noise conditions (Normalized Root Mean Square Error of 1.3 % at SNR = 0 dB) while spending a significantly less computational time (0.054 s). The network also does not require a large scale dataset during training, thus making it ideal for applications with limited data that require fast and accurate phase unwrapping.