Documents
Presentation Slides
Presentation Slides
Large-scale ASR Domain Adaptation using Self- and Semi-supervised Learning
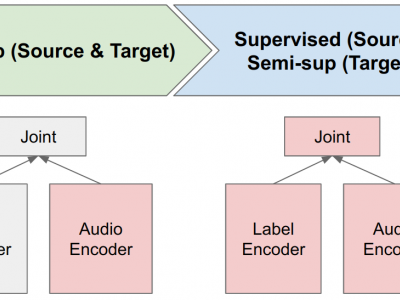
- Citation Author(s):
- Submitted by:
- Dongseong Hwang
- Last updated:
- 4 May 2022 - 9:06pm
- Document Type:
- Presentation Slides
- Document Year:
- 2022
- Event:
- Presenters:
- Dongseong Hwang
- Paper Code:
- SPE-22.1
- Categories:
- Log in to post comments
Self- and semi-supervised learning methods have been actively investigated to reduce labeled training data or enhance the model performance. However, the approach mostly focus on in-domain performance for public datasets. In this study, we utilize the combination of self- and semi-supervised learning methods to solve unseen domain adaptation problem in a large-scale production setting for online ASR model. This approach demonstrates that using the source domain data with a small fraction of the target domain data (3%) can recover the performance gap compared to a full data baseline: relative 13.5% WER improvement for target domain data.
Links: