Documents
Presentation Slides
MaskCycleGAN-VC: Learning Non-parallel Voice Conversion with Filling in Frames
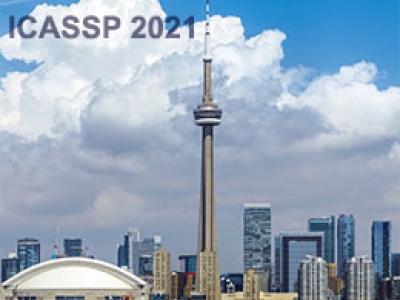
- Citation Author(s):
- Submitted by:
- Takuhiro Kaneko
- Last updated:
- 29 June 2021 - 7:18am
- Document Type:
- Presentation Slides
- Document Year:
- 2021
- Event:
- Presenters:
- Takuhiro Kaneko
- Paper Code:
- SPE-11.1
- Categories:
- Log in to post comments
Non-parallel voice conversion (VC) is a technique for training voice converters without a parallel corpus. Cycle-consistent adversarial network-based VCs (CycleGAN-VC and CycleGAN-VC2) are widely accepted as benchmark methods. However, owing to their insufficient ability to grasp time-frequency structures, their application is limited to mel-cepstrum conversion and not mel-spectrogram conversion despite recent advances in mel-spectrogram vocoders. To overcome this, CycleGAN-VC3, an improved variant of CycleGAN-VC2 that incorporates an additional module called time-frequency adaptive normalization (TFAN), has been proposed. However, an increase in the number of learned parameters is imposed. As an alternative, we propose MaskCycleGAN-VC, which is another extension of CycleGAN-VC2 and is trained using a novel auxiliary task called filling in frames (FIF). With FIF, we apply a temporal mask to the input mel-spectrogram and encourage the converter to fill in missing frames based on surrounding frames. This task allows the converter to learn time-frequency structures in a self-supervised manner and eliminates the need for an additional module such as TFAN. A subjective evaluation of the naturalness and speaker similarity showed that MaskCycleGAN-VC outperformed both CycleGAN-VC2 and CycleGAN-VC3 with a model size similar to that of CycleGAN-VC2. Audio samples are available at https://www.kecl.ntt.co.jp/people/kaneko.takuhiro/projects/maskcyclegan-vc/.