Documents
Presentation Slides
A MULTI-PERSPECTIVE APPROACH TO ANOMALY DETECTION FOR SELF-AWARE EMBODIED AGENTS
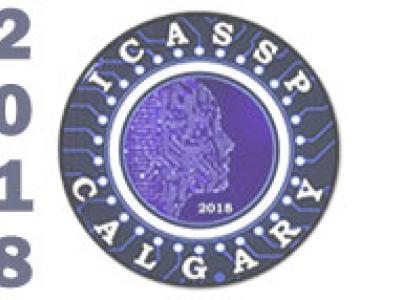
- Citation Author(s):
- Submitted by:
- Lucio Marcenaro
- Last updated:
- 18 April 2018 - 10:40am
- Document Type:
- Presentation Slides
- Document Year:
- 2018
- Event:
- Presenters:
- Lucio Marcenaro
- Paper Code:
- SS-L2.5
- Categories:
- Log in to post comments
This paper focuses on multi-sensor anomaly detection for moving cognitive agents using both external and private first-person visual observations. Both observation types are used to characterize agents’ motion in a given environment. The proposed method generates locally uniform motion models by dividing a Gaussian process that approximates agents’ displacements on the scene and provides a Shared Level (SL) self-awareness based on Environment Centered (EC) models. Such models are then used to train in a semi-unsupervised way a set of Generative Adversarial Networks (GANs) that produce an estimation of external and internal parameters of moving agents. Obtained results exemplify the feasibility of using multi-perspective data for predicting and analyzing trajectory information.