Documents
Presentation Slides
NEUROMORPHIC VISION SENSING FOR CNN-BASED ACTION RECOGNITION
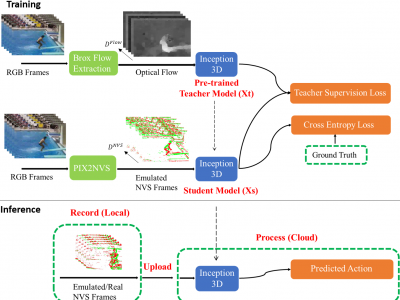
- Citation Author(s):
- Submitted by:
- Aaron Chadha
- Last updated:
- 10 May 2019 - 9:31am
- Document Type:
- Presentation Slides
- Document Year:
- 2019
- Event:
- Presenters:
- Aaron Chadha
- Paper Code:
- 3575
- Categories:
- Log in to post comments
Neuromorphic vision sensing (NVS) hardware is now gaining traction as a low-power/high-speed visual sensing technology that circumvents the limitations of conventional active pixel sensing (APS) cameras. While object detection and tracking models have been investigated in conjunction with NVS, there is currently little work on NVS for higher-level semantic tasks, such as action recognition. Contrary to recent work that considers homogeneous transfer between flow domains (optical flow to motion vectors), we propose to embed an NVS emulator into a multi-modal transfer learning framework that carries out heterogeneous transfer from optical flow to NVS. The potential of our framework is showcased by the fact that, for the frst time, our NVS-based results achieve comparable action recognition performance to motion-vector or opticalflow based methods (i.e., accuracy on UCF-101 within 8.8% of I3D with optical flow), with the NVS emulator and NVS camera hardware offering 3 to 6 orders of magnitude faster frame generation (respectively) compared to standard Brox optical flow. Beyond this signifcant advantage, our CNN processing is found to have the lowest total GFLOP count against all competing methods (up to 7.7 times complexity saving compared to I3D with optical flow).